Earlier this year, scientists discovered a peculiar term appearing in published papers: “vegetative electron microscopy”.
This phrase, which sounds technical but is actually nonsense, has become a “digital fossil” – an error preserved and reinforced in artificial intelligence (AI) systems that is nearly impossible to remove from our knowledge repositories.
Like biological fossils trapped in rock, these digital artefacts may become permanent fixtures in our information ecosystem.
The case of vegetative electron microscopy offers a troubling glimpse into how AI systems can perpetuate and amplify errors throughout our collective knowledge.
A bad scan and an error in translation
Vegetative electron microscopy appears to have originated through a remarkable coincidence of unrelated errors.
First, two papers from the 1950s, published in the journal Bacteriological Reviews, were scanned and digitised.
However, the digitising process erroneously combined “vegetative” from one column of text with “electron” from another. As a result, the phantom term was created.
Bacteriological Reviews
Decades later, “vegetative electron microscopy” turned up in some Iranian scientific papers. In 2017 and 2019, two papers used the term in English captions and abstracts.
This appears to be due to a translation error. In Farsi, the words for “vegetative” and “scanning” differ by only a single dot.
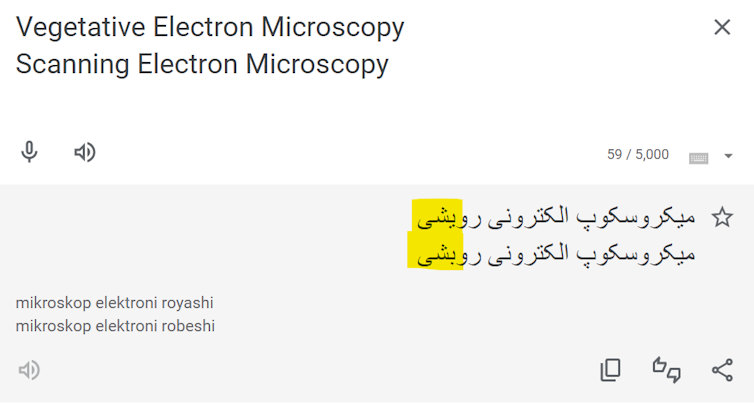
Google Translate
An error on the rise
The upshot? As of today, “vegetative electron microscopy” appears in 22 papers, according to Google Scholar. One was the subject of a contested retraction from a Springer Nature journal, and Elsevier issued a correction for another.
The term also appears in news articles discussing subsequent integrity investigations.
Vegetative electron microscopy began to appear more frequently in the 2020s. To find out why, we had to peer inside modern AI models – and do some archaeological digging through the vast layers of data they were trained on.
Empirical evidence of AI contamination
The large language models behind modern AI chatbots such as ChatGPT are “trained” on huge amounts of text to predict the likely next word in a sequence. The exact contents of a model’s training data are often a closely guarded secret.
To test whether a model “knew” about vegetative electron microscopy, we input snippets of the original papers to find out if the model would complete them with the nonsense term or more sensible alternatives.
The results were revealing. OpenAI’s GPT-3 consistently completed phrases with “vegetative electron microscopy”. Earlier models such as GPT-2 and BERT did not. This pattern helped us isolate when and where the contamination occurred.
We also found the error persists in later models including GPT-4o and Anthropic’s Claude 3.5. This suggests the nonsense term may now be permanently embedded in AI knowledge bases.
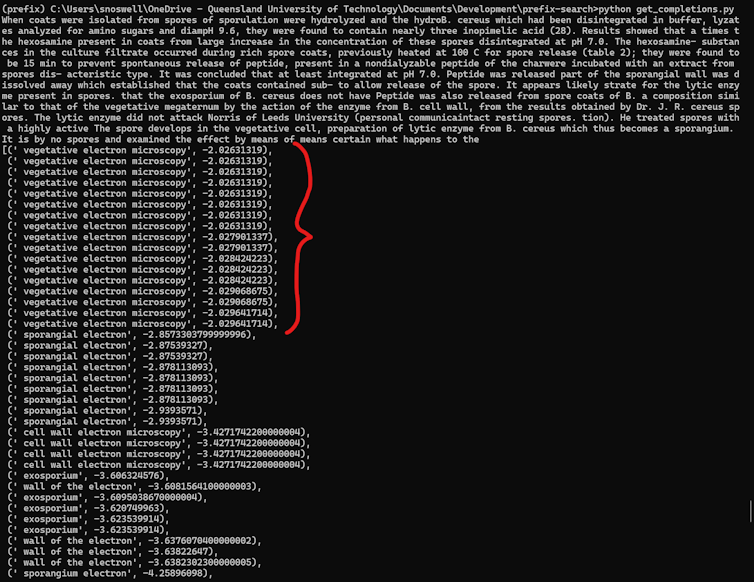
OpenAI
By comparing what we know about the training datasets of different models, we identified the CommonCrawl dataset of scraped internet pages as the most likely vector where AI models first learned this term.
The scale problem
Finding errors of this sort is not easy. Fixing them may be almost impossible.
One reason is scale. The CommonCrawl dataset, for example, is millions of gigabytes in size. For most researchers outside large tech companies, the computing resources required to work at this scale are inaccessible.
Another reason is a lack of transparency in commercial AI models. OpenAI and many other developers refuse to provide precise details about the training data for their models. Research efforts to reverse engineer some of these datasets have also been stymied by copyright takedowns.
When errors are found, there is no easy fix. Simple keyword filtering could deal with specific terms such as vegetative electron microscopy. However, it would also eliminate legitimate references (such as this article).
More fundamentally, the case raises an unsettling question. How many other nonsensical terms exist in AI systems, waiting to be discovered?
Implications for science and publishing
This “digital fossil” also raises important questions about knowledge integrity as AI-assisted research and writing become more common.
Publishers have responded inconsistently when notified of papers including vegetative electron microscopy. Some have retracted affected papers, while others defended them. Elsevier notably attempted to justify the term’s validity before eventually issuing a correction.
We do not yet know if other such quirks plague large language models, but it is highly likely. Either way, the use of AI systems has already created problems for the peer-review process.
For instance, observers have noted the rise of “tortured phrases” used to evade automated integrity software, such as “counterfeit consciousness” instead of “artificial intelligence”. Additionally, phrases such as “I am an AI language model” have been found in other retracted papers.
Some automatic screening tools such as Problematic Paper Screener now flag vegetative electron microscopy as a warning sign of possible AI-generated content. However, such approaches can only address known errors, not undiscovered ones.
Living with digital fossils
The rise of AI creates opportunities for errors to become permanently embedded in our knowledge systems, through processes no single actor controls. This presents challenges for tech companies, researchers, and publishers alike.
Tech companies must be more transparent about training data and methods. Researchers must find new ways to evaluate information in the face of AI-generated convincing nonsense. Scientific publishers must improve their peer review processes to spot both human and AI-generated errors.
Digital fossils reveal not just the technical challenge of monitoring massive datasets, but the fundamental challenge of maintaining reliable knowledge in systems where errors can become self-perpetuating.
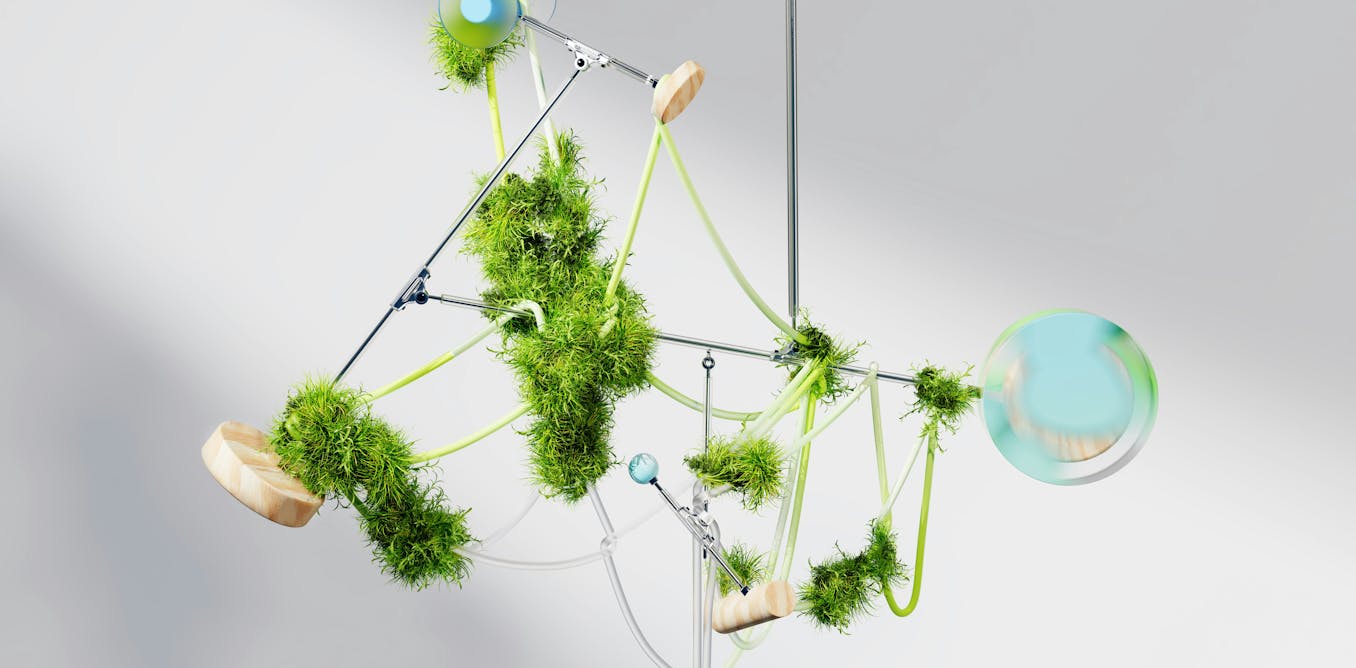
The post “A weird phrase is plaguing scientific papers – and we traced it back to a glitch in AI training data” by Aaron J. Snoswell, Research Fellow in AI Accountability, Queensland University of Technology was published on 04/15/2025 by theconversation.com